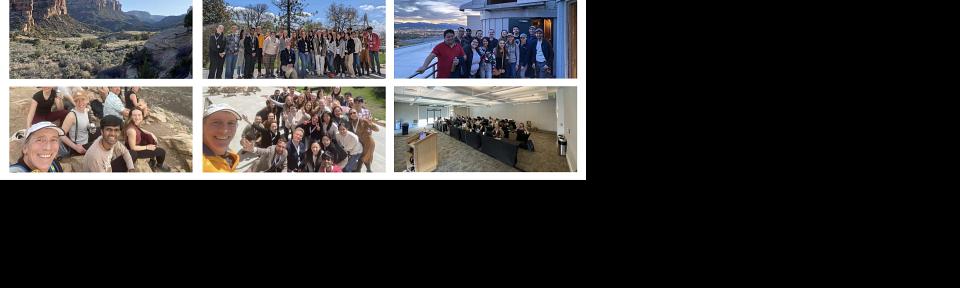

Course Syllabus
Course Syllabus
April 7 - 18
The course will be held in Salt Lake City, UT. The days will be comprised of live lectures and interactive computer labs. We will provide an excellent and personalized learning experience. Each day will start with an introduction by course Co-Directors Robert Welsh and Anna Docherty, along with the highlight lecturer of that day.
The days are broken into a series of lectures paired with practical computer labs. Each lecture will be 45-55 minutes in length, followed by a short break, and then a related practical computer lab exercise. Each day will end with an open discussion. Lectures will facilitate interaction between instructors and course attendees. Each day of the course is expected to last 7 hours, with the exception of Day 6, which will be a half day.
Prior to the start of the course, you will be connected with course instructors to ensure you have the proper computational resources installed.
- Software validation
- Statistics Primer and Linear Regression
- Maximum Likelihood, General Linear Model
- Scientific Rigor and Transparency
- Responsible Conduct of Research
- Mixed and Random Effects Models
- Introduction to Longitudinal Modeling
- Efficient Longitudinal Modeling
- Introduction to Bayesian Modeling
- Spatial Priors
- Empirical Population Priors
- Latent Variables (e.g. diagnosis)
- Modeling Heritability
- SNP-based Heritability and Introduction to GWAS
- Using Data from GWAS
- Polygenic Risk Scoring with Clumping and Thresholding
- Polygenic Risk Scoring in Bayesian Framework
- Extended Applications of Statistical Genetics
We will have a guest lecture and brunch. This will be followed by a short walk to the Natural History Museum of Utah where you will have a ticket to wander about the exhibits.
Those interested in a hike can join as we hike along the Bonneville Shoreline Trail in the later afternoon.
- Network Science Background, Basic Measures
- Networks in Neuroscience
- Scientific Rigor and Transparency
- Modular Structure of Brain Networks
- Structure-Function Relationships
- Biophysical Models and Stylized Models
- Cross Validating Models
- Neuroimaging: Properties and Benefits of Fusion
- Sequential vs Simultaneous Acquisition
- Symmetric vs Asymmetric Analysis, Joint Models
- Open Discussion: Benefits and Pitfalls (with Docherty and Welsh)
- Predictive Models: Why They Work
- Predictive Models and Criteria for a Good Algorithm
- Building Biomarkers: Problems and Translational Neuroimaging 2.0
- Time is reserved in the morning for open access to computer labs with instructors
- Continuing Education Demonstration
- Mini Lab
- Group discussion about current research and grant planning, with career mentorship
- Course Summary
Course Computer Labs
- R/Matlab/Bash Intro Data
- Means, Variances, and Covariances
- Matrices, PCA
- Distributions
- Bayes Primer
- Central Limit Theorem
- Linear Regression
- Maximum Likelihood Estimation
- Introduction to Bayes
- ACE Model
- SEM estimation of AVE Model
- SEM, two phenotypes
- Genotype data, Plink file format
- PCA, Ancestry estimation
- GWAS, Genome wide association study
- SNP heritability and genetic correlation
- Structure and Function
- Communication models
- Neural Mass Models
- Linear Mixed Models
- Longitudinal Data Analysis Part 1
- Longitudinal Data Analysis Part 2
- PRSice polygenic risk scoring
- PRScs polygenic risk scoring
- Online resources for genetic analysis
- Ridge/Lasso
- SVM and ROC
- Modeling Example: Pain Prediction
- Bayesian Single-Subject "Template" ICA
- Bayes fMRI
- Network Visualization
- Small worlds, hubs, and rich cubs
- Communities and Modules
- Introduction to Canonical Correlation Analysis