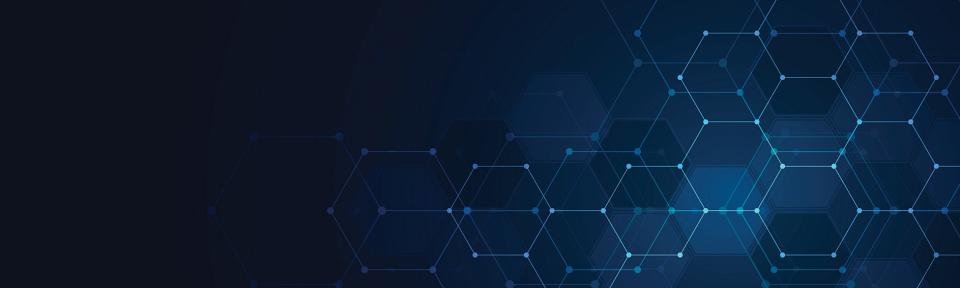
Artificial Intelligence in Oncology
As advances in artificial intelligence (AI) continue to reshape the landscape of healthcare, this workshop aims to explore the transformative potential of AI in the field of cancer research and treatment. The integration of AI technologies presents unprecedented opportunities to enhance early detection, precision medicine, and personalized treatment strategies for cancer patients. This session will delve into the machine learning (ML) based models identifying novel biomarkers, predicting cancer risk as well drug resistance using multi- modal data including EHR, multi-omics and imaging. By bringing together experts from both the AI and cancer research communities, this session aims to foster collaborative efforts, share insights, and pave the way for a future where AI plays a pivotal role in advancing cancer care.
Scope
In the rapidly evolving landscape of healthcare, the integration of artificial intelligence (AI) stands as a beacon of transformative potential, promising to advance patient care, diagnosis, and treatment methodologies. This workshop will include the AI applications in oncology, fostering innovation and improving patient outcomes. Participants will learn about the latest AI advancements and real-world applications in cancer diagnostics, treatment and prognosis. Through expert-led sessions, and collaborative learning, attendees will navigate the latest AI methods in oncology. The workshop is designed for a broad audience, including healthcare professionals, researchers, students, and anyone interested in the intersection of AI and oncology.
TOPICS
|
|
|
|
|
|
|
PROGRAM
Time |
Format |
Topic |
Speakers |
5 Minutes |
Podium Presentation |
Introduction & Keynote |
|
30 Minutes |
Podium Presentation |
Opening Keynote |
TBD |
40 Minutes |
Research presentations |
Various research topics |
Communication author of 3 to 4 accepted abstract/papers |
15-20 Minutes |
Break |
Connect and collaborate |
N/A
|
30 Minutes |
Podium Presentation |
Middle Keynote |
Ece Uzun |
40 Minutes |
Research presentations |
Various research topics |
Communication author of 3 to 4 accepted abstract/papers |
30 Minutes |
Podium Talk |
Closing Keynote |
TBD
|
Committee

Zhongming Zhao, PhD

Qianqian Song, PhD

Guangyu Wang, Phd

AIk Chan (AC) Tan, PhD