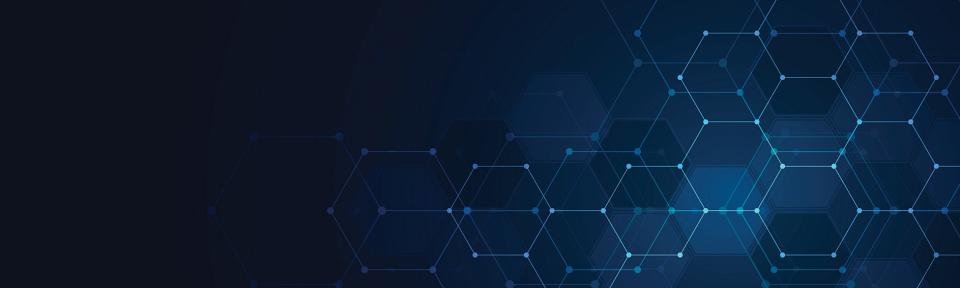
Program - AI Reliable
Program
Tuesday, July 9th | 1:45 - 6 pm
Time |
Format |
Topic |
Speakers |
10 minutes |
Podium presentation |
Welcome Address |
Linying Zhang |
50 Minutes |
Podium presentation |
Opening Keynote |
George Hripcsak |
60 minutes |
Research presentation |
Spotlight presentation |
Authors of 4 accepted papers |
15 minutes |
Coffee Break |
Connect and collaborate |
N/A |
80 minutes |
Podium presentation |
4 Rising Star Presentations on AI in Medicine |
Michael Oberst, Zhiyu Wan, Vicky Tiase, Laura Wiley |
20 minutes |
Panel |
Reflection Panel on Real-World Evidence with AI |
George Hripcsak, Scott L. Duvall, David K. Vawdrey, Adam Wilcox, Linying Zhang |
20 minutes |
Podium presentation |
Closing Keynote |
Scott L. Duvall |
Spotlight Presentations
Federated Learning of Medical Concepts Embedding using BEHRT - Ofir Ben Shoham and Nadav Rappoport
A Formal Comparison of a Large Language Model Chatbot to Natural Language Processing for Concept Extraction from Medical Text Reports - Adam Wilcox, Trudy Landreth, Joshua Denzer, Patrick Donohue, Navya Bhagat and Matthew Schuelke
Enhancing Early Gestational Diabetes Prediction with Imputation - Leyao Ma, Lin Yang, Yaxin Wang, Jie Hao, Liangkun Ma, Ziyang Wang, Yin Sun and Jiao Li
Evaluating Generative AI’s Ability to Identify Cancer Subtypes in Publicly Available Structured Genetic Datasets - Ethan Hillis, Kriti Bhattarai and Zachary Abrams
Rising Stars

Zhiyu Wan, PhD
Zhiyu Wan, PhD, is a postdoctoral research fellow in the Department of Biomedical Informatics, Vanderbilt University Medical Center. He is mentored by Dr. Bradley A. Malin, and is currently working in the Health Information Privacy Laboratory (HIPLAB), and the Center for Genetic Privacy and Identity in Community Settings (GetPreCiSe). His research interests include: optimizing privacy-preserving data sharing, with an emphasis on health and genomic data; leveraging game theory, artificial intelligence, and machine learning techniques to improve social good; and analyzing social networks and network security. His latest research project is using game theory to protect genomic data against privacy attacks. He finished his PhD in Computer Science at the Department of Electrical Engineering and Computer Science, School of Engineering, Vanderbilt University. He obtained his bachelor’s degree in Automation (minor in Business Administration), as an outstanding graduate, from Xi’an Jiaotong University, which he entered through the Special Class for the Gifted Young of China.
Title: Promoting responsive real world data sharing in medicine using AI agents
Abstract: In the latest AI boom, effective deep learning and large language models that rely upon gigantic amounts of training data are accelerating healthcare and medicine research. An insufficient amount of real-world data can lead to unreliable and unfair machine-learning models. However, sharing health data has privacy concerns. Years ago, I started working on optimizing the way to share real-world data responsively, with a focus on promoting privacy and fairness. Using a game theoretic lens, I found the best way to share genomic data, electronic health records (EHR), images, and voice recordings from patients to third parties or the public. In most experiments, I analyzed privacy risk and data utility using real-world data from EHR along with external resources such as voter registration lists and recreational genomic databases. This is a fundamentally different approach because it accounts for adversarial behavior and capabilities and tailors protections to anticipated recipients with reasonable resources, not adversaries with unlimited means. This multi-agent-based approach can be integrated with existing data protection models and can be applied to assess risk and suggest an optimal data-sharing strategy based on a privacy-utility tradeoff. The results show that most data can be effectively shared with low privacy risk in a game theoretic model. Additionally, I used these risk metrics to evaluate and guide the development of data protection methods such as synthetic data generation which generates fake health records based on real world data and is expected to bring real-world evidence in medicine.

Michael Oberst, PhD
Michael Oberst is an assistant professor of computer science and a member of the Malone Center for Engineering in Healthcare. Dr. Oberst’s research focuses on reliable machine learning for decision-making in health care. His long-term goal is to ensure that machine learning systems are as reliable as any FDA-approved medication or diagnostic test. His work has been published in machine learning conferences such as NeurIPS, ICML, and AISTATS; his research has additionally appeared in Science Translational Medicine. Dr. Oberst received a BS in statistics from Harvard University and a PhD in computer science from MIT. Prior to joining Johns Hopkins, he was a postdoctoral associate in the Machine Learning Department at Carnegie Mellon University.
Title: Auditing Fairness under Unobserved Confounding
Abstract: Inequity in resource allocation has been well-documented in many domains, such as healthcare. Causal measures of equity / fairness seek to isolate biases in allocation that are not explained by other factors, such as underlying need. However, these fairness measures require the (strong) assumption that we observe all relevant indicators of need, an assumption that rarely holds in practice. For instance, if resources are allocated based on indicators of need that are not recorded in our data ("unobserved confounders"), we may understate (or overstate) the amount of inequity. In this talk, I will present work demonstrating that we can still give informative bounds on certain causal measures of fairness, even while relaxing (or even eliminating) the assumption that all relevant indicators of need are observed. We use the fact that in many real-world settings (e.g., the release of a new treatment) we have data from prior to any allocation, which can be used to derive unbiased estimates of need. This result is of immediate practical interest: we can audit unfair outcomes of existing decision-making systems in a principled manner. For instance, in a real-world study of Paxlovid allocation, we show that observed racial inequity cannot be explained by unobserved confounders of the same strength as important observed covariates. The preprint is available at https://arxiv.org/abs/2403.14713

Victoria Tiase, PhD, RN-BC, FAMIA, FNAP, FAAN
Dr. Tiase, a nurse informaticist, is an Assistant Professor of Biomedical Informatics and Nursing at the University of Utah. Her expertise ranges from leading EHR implementations, integrating patient-generated health data, to mentoring digital health startups. Dr. Tiase serves on the boards of the Alliance for Nursing Informatics, AMIA, and Friends of NINR. She was appointed as the informatics expert to the National Academy of Medicine’s Future of Nursing 2030 Committee to envision the nurse’s role in using technology to tackle disparities, promote health equity, and create healthier communities. Her current research involves the computational modeling of nursing workload to improve the systems, structures, and policies that support a diverse nursing workforce.
Title: Developing a Logical Data Model for Nursing Workload
Abstract: Gathering sufficient data that are reliable, reproducible, generalizable, and that represent nursing contributions within the context of work activities remains a complex, unsolved problem. Advances in informatics and electronic health record audit log data have shown promise in measuring clinician work activities. To effectively use these approaches, it is necessary to structure the raw audit logs into a consistent and scalable logical data model that can be consumed by machine learning algorithms. We aimed to conceptualize a logical data model for nurse-EHR interactions that would support the future development of temporal ML models based on EHR audit log data.

Laura Wiley, PhD, MS
Dr. Wiley develops methods for using data from electronic health records for precision medicine discovery and implementation. She is a an Associate Professor in the Department of Biomedical Informatics, and Chief Data Scientistic of Health Data Compass at the University of Colorado Anschutz Medical Campus. She completed undergraduate degrees in chemistry and molecular biology at the University of Denver followed by a Masters in biomedical informatics (2010) and a PhD in human genetics at Vanderbilt University (2016). Her published work involves developing computational phenotyping algorithms for use in EHR-linked biobanks and investigating new algorithms for precision dosing of warfarin in African Americans. Her current work focuses on clinical evidence generation from electronic health records and informatics implementation, serving as the lead informatician on an NIH Cancer Moonshot-funded project to create a comprehensive tobacco cessation service at the University of Colorado Cancer Center. Dr. Wiley is actively involved in the informatics community, currently serving on the editorial board of the Journal of Biomedical Informatics. She Chaired the 2022 AMIA Informatics Summit and has served as Vice-Chair of the American Medical Informatics Association (AMIA) 2019 and 2023 Annual Symposiums and the 2021 Informatics Summit. She also has served as Chair of AMIA Working Group Steering Committee, and an ex officio member of the AMIA Board of Directors. Further she has co-developed the Coursera Clinical Data Science Specialization - a series of six MOOCs designed to teach clinical research informatics skills which has been taken by nearly 36,000 students across the globe.
Title: TBA
Abstract: TBA
SCIENTIFIC PAPER PROGRAM COMMITTEE

Peter Rijnbeek, PhD

Mattia Prosperi, PhD

Xia Ning, PhD
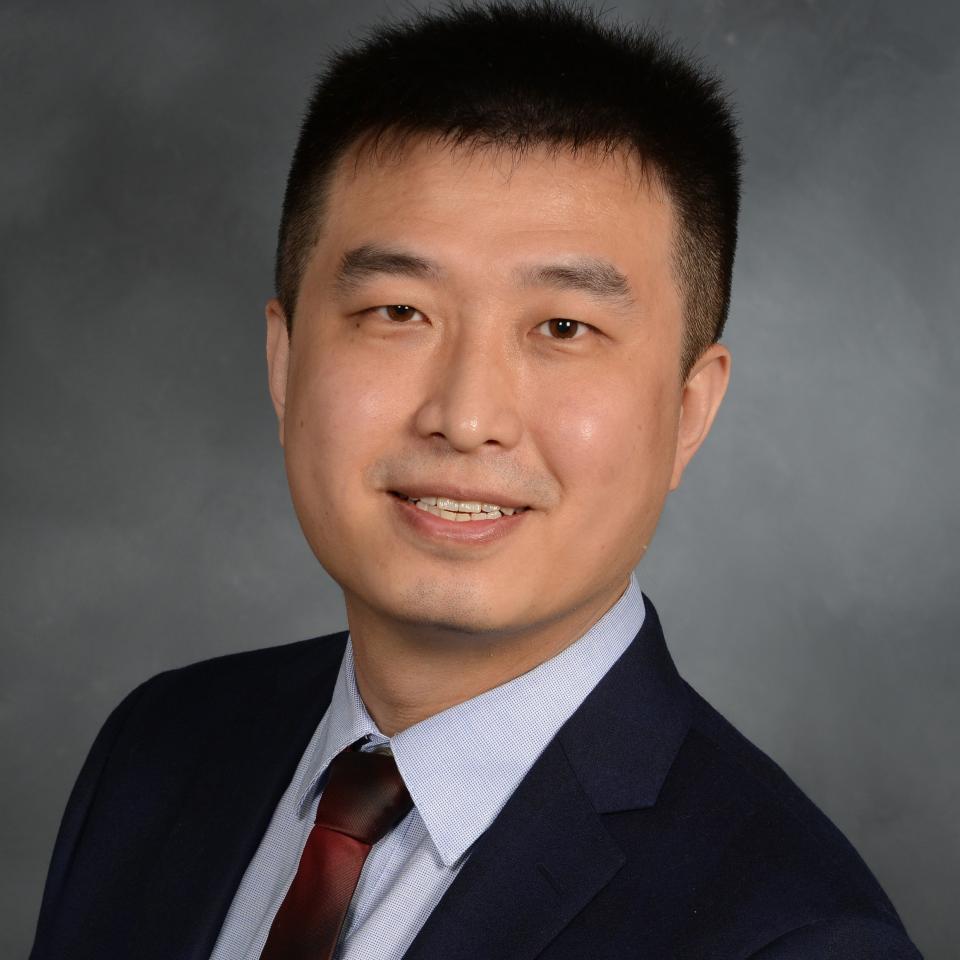
Yifan Peng, PhD
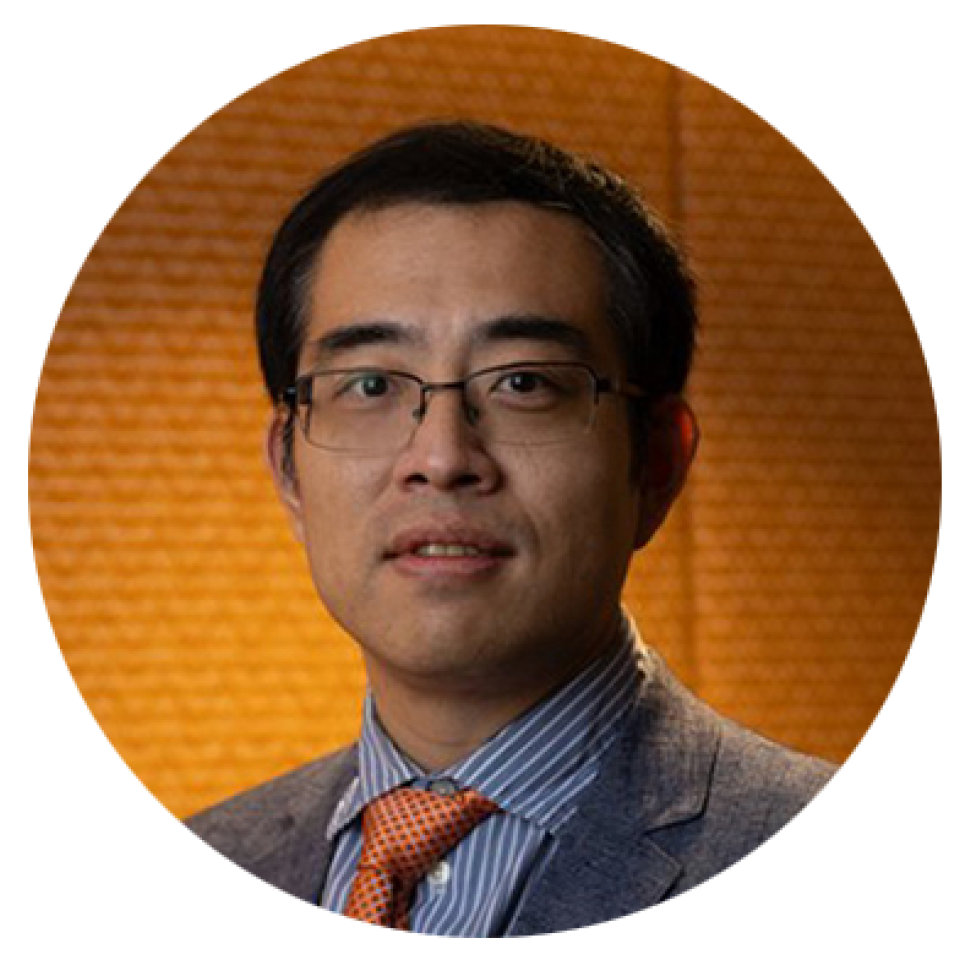
Xiaoqian Jiang, PhD

Larry Han, PhD

Thomas Reese, PharmD, PhD