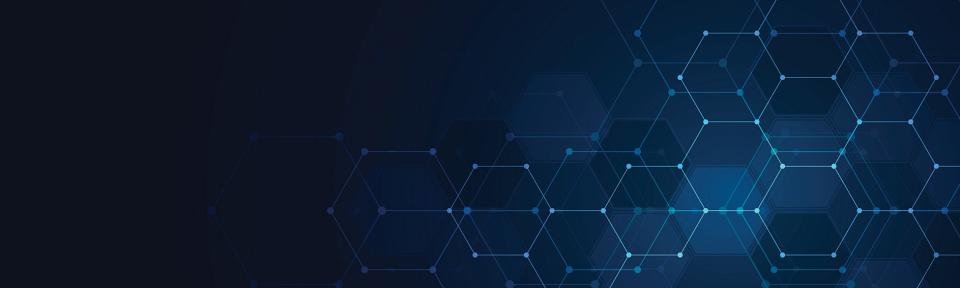
AI for Reliable and Equitable Real World Evidence Generation in Medicine
The "AI for Reliable and Equitable Real World Evidence Generation in Medicine" workshop is dedicated to advancing the understanding and exploring the transformative role of artificial intelligence (AI) in analyzing real-world data (RWD) for real-world evidence (RWE) generation, leading to evidence-based medicine (EBM). Focused on leading-edge research and innovation, the workshop will feature research papers and panel discussions that delve into key aspects of machine learning innovations and applications in RWE generation from EHRs and claims, including structured data, natural language processing (NLP) of clinical notes, medical imaging, and waveform data processing from wearable devices. The workshop will feature both innovative AI methodology as well as their applications to real-world problems and their impact on transforming evidence-based medicine. The workshop seeks to facilitate in-depth discussions on the integration of AI technologies to enhance the reliability and equity of RWE generation. The workshop serves as a platform for engaging multiple stakeholders across healthcare research, including researchers, clinicians, pharmaceutical and industry professionals to delve into the intricacies of these advanced methodologies, fostering dialogue and collaboration. Attendees can anticipate in-depth discussions, presentations, and networking opportunities, gaining valuable insights into the forefront of AI-driven strategies shaping the future of these discoveries.
SCOPE
AI encompasses statistical and computational machine learning, deep learning, and generative AI (e.g., Large Language Model, Diffusion Models, etc), all are welcomed approaches. We include innovative AI methods as well as application of AI methods to the field of evidence generation for real-world effectiveness, safety, and equity research.
TOPICS
|
|
|
|
|
|
|
PROGRAM FORMAT
Time |
Format |
Topic |
Speakers |
10 minutes |
Podium presentation |
Welcome Address |
Linying Zhang |
50 Minutes |
Podium presentation |
Opening Keynote |
George Hripcsak |
60 minutes |
Research presentation |
Spotlight presentation |
Authors of 4 accepted papers |
15 minutes |
Coffee Break |
Connect and collaborate |
N/A |
80 minutes |
Podium presentation |
4 Rising Star Presentations on AI in Medicine |
Michael Oberst, Zhiyu Wan, Vicky Tiase, Laura Wiley |
20 minutes |
Panel |
Reflection Panel on Real-World Evidence with AI |
George Hripcsak, Scott L. Duvall, David K. Vawdrey, Adam Wilcox, Linying Zhang |
20 minutes |
Podium presentation |
Closing Keynote |
Scott L. Duvall |
Keynote Speakers
Opening Keynote

George Hripcsak, MD, MS
Dr. George Hripcsak, MD, MS, is interested in the clinical information stored in electronic health records and in the development of next-generation health record systems. Health record data are sparse, irregularly sampled, complex, and biased. Using nonlinear time series analysis methods borrowed from statistical physics, machine learning, knowledge engineering, and natural language processing, he is developing the methods necessary to support clinical research and patient safety initiatives using health record data. Dr. Hripcsak also has a long track record of developing, implementing, and studying informatics interventions to improve health care. He has published frequently on evaluation in biomedical informatics, leveraging his training in biostatistics. Dr. Hripcsak is Vivian Beaumont Allen Professor at Columbia University’s Department of Biomedical Informatics. He is a board-certified internist with degrees in chemistry, medicine, and biostatistics. He leads the Observational Health Data Sciences and Informatics (OHDSI) coordinating center; OHDSI is an international network with thousands of collaborators and health records on almost one billion patients. In precision medicine, he serves as a PI on Columbia’s eMERGE grant, Columbia’s regional recruitment center for the All of Us Research Program, and Columbia’s role on the All of Us Data and Research Center. He co-chaired the Meaningful Use Workgroup of U.S. Department of Health and Human Services’s Office of the National Coordinator of Health Information Technology. Dr. Hripcsak is a member of the National Academy of Medicine, the American College of Medical Informatics, the International Academy of Health Sciences Informatics, and the New York Academy of Medicine. He was awarded the 2022 Morris F. Collen Award of Excellence by the American College of Medical Informatics. He has over 500 publications.
Closing Keynote

Scott L. DuVall, PhD
Driven by a passion for leveraging data to make a meaningful impact, Scott L. DuVall, PhD, continues to push the boundaries of medical informatics and data science to benefit Veterans and the broader medical community. Dr. DuVall serves as the Director of the Department of Veterans Affairs (VA) Informatics and Computing Infrastructure (VINCI), a resource center that offers secure computing environments, analytic tools, support services, and a nation-wide view of VA electronic medical record and administrative claims data to more than 10,000 VA-credentialed researchers. Dr. DuVall spearheads the VA Office of Research and Development (ORD) initiative to "Put VA Data to Work for Veterans" that encompasses curating and standardizing data, linking and integrating external data sources, extracting clinical, social, and demographic information using natural language processing, and developing and implementing rigorous methods for reliable real-world evidence. Under his leadership, the VINCI team puts these data to work to enhance Veteran health by leading, enabling, and supporting clinical decision making and scientific discovery. Dr. DuVall is a Professor in the University of Utah School of Medicine within the Department of Internal Medicine Division of Epidemiology. His research focuses on the development and application of informatics tools and advanced analytical methods on electronic health care data in pharmacoepidemiology and outcomes research. He also directs a federally certified service recharge center within the University of Utah Health Science Core Facilities that offers data science and advanced analysis, computer-aided medical record review, natural language processing, and software development services. Dr. DuVall received a Bachelor of Science in Computer Science and a Doctor of Philosophy in Biomedical Informatics.
RISING STARS
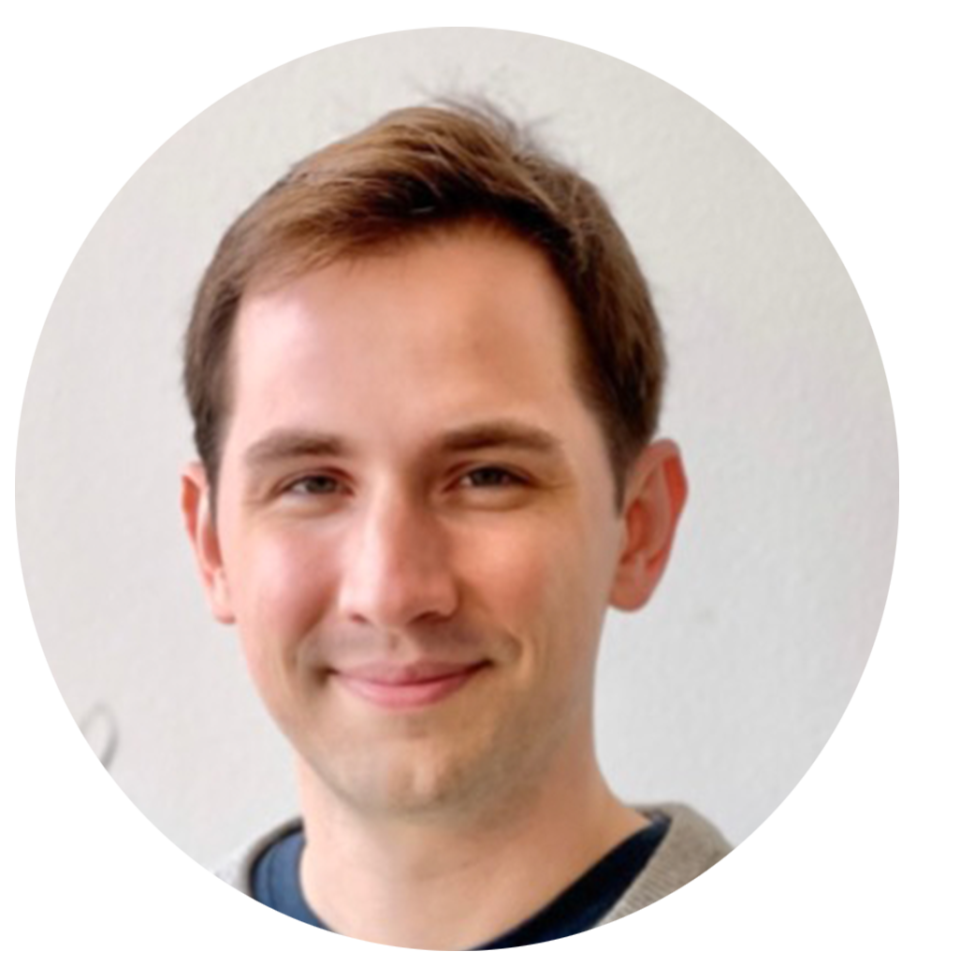
Michael Oberst, PhD

Zhiyu Wan, PhD
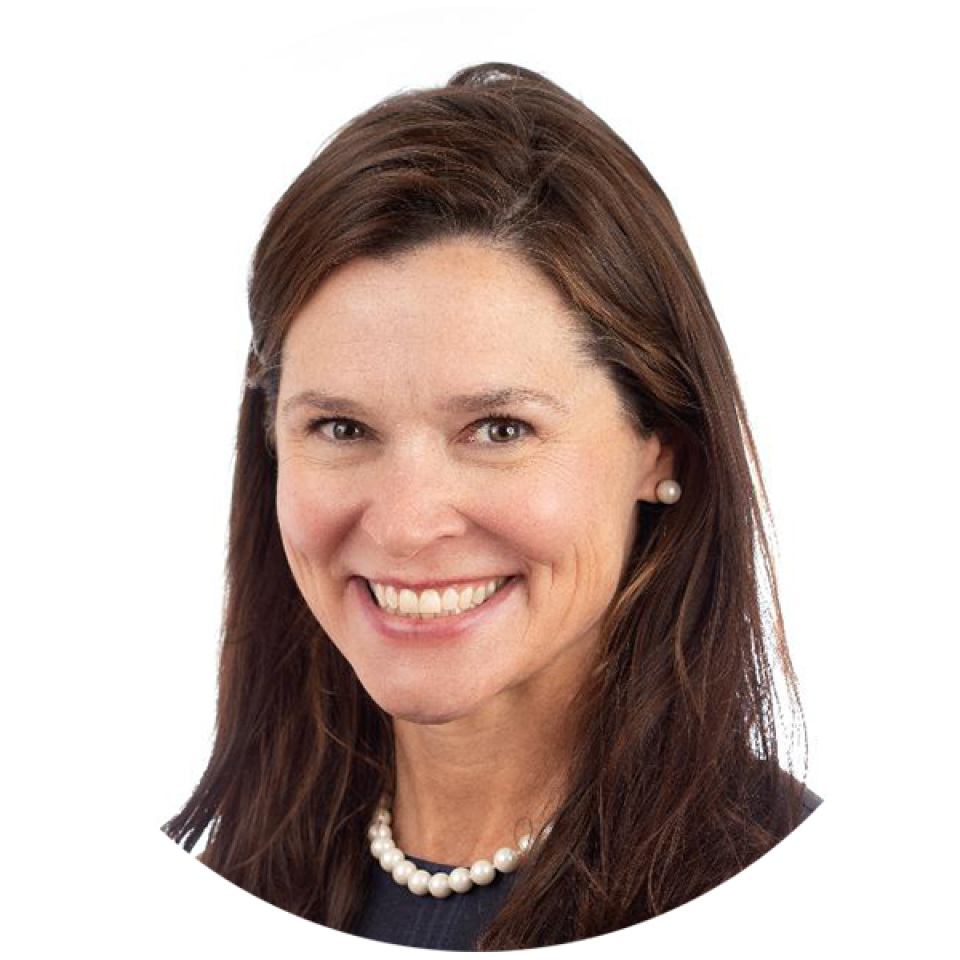
Victoria Tiase, PhD, RN-BC, FAMIA, FNAP, FAAN

Laura Wiley, PhD, MS
Panelists
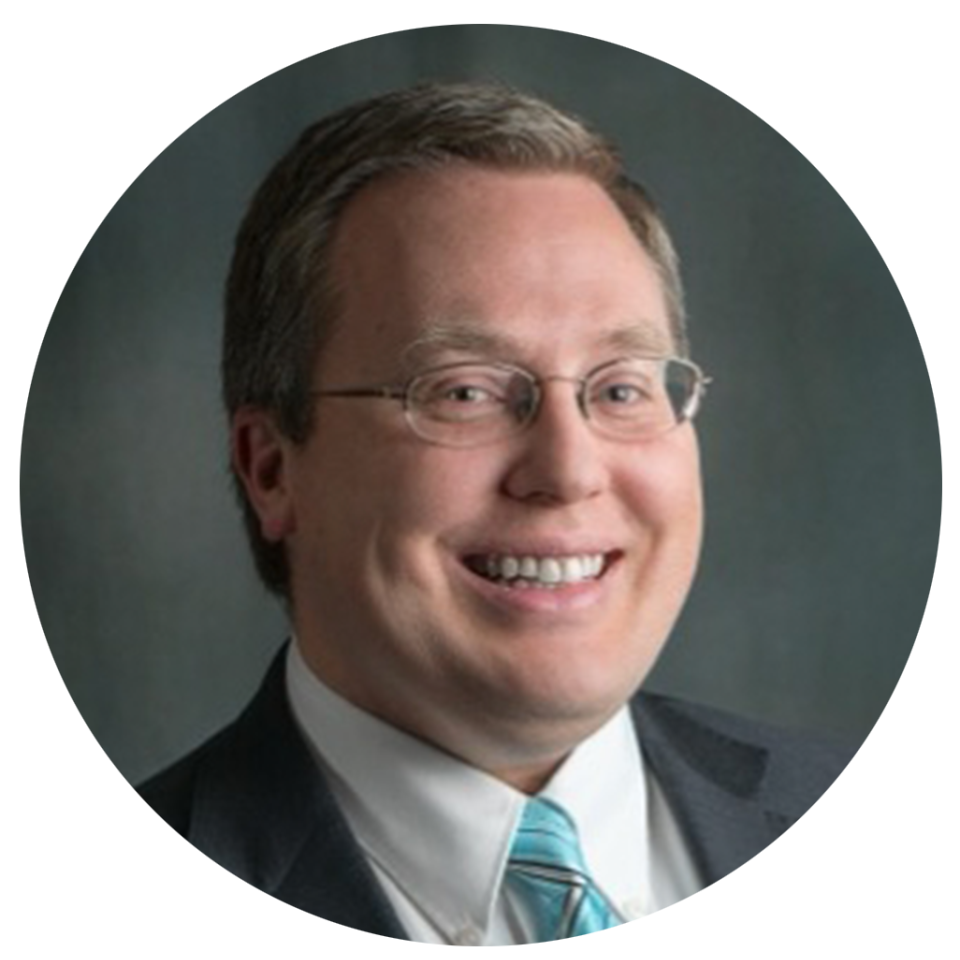
Scott L. DuVall, PhD

George Hripcsak, MD, MS
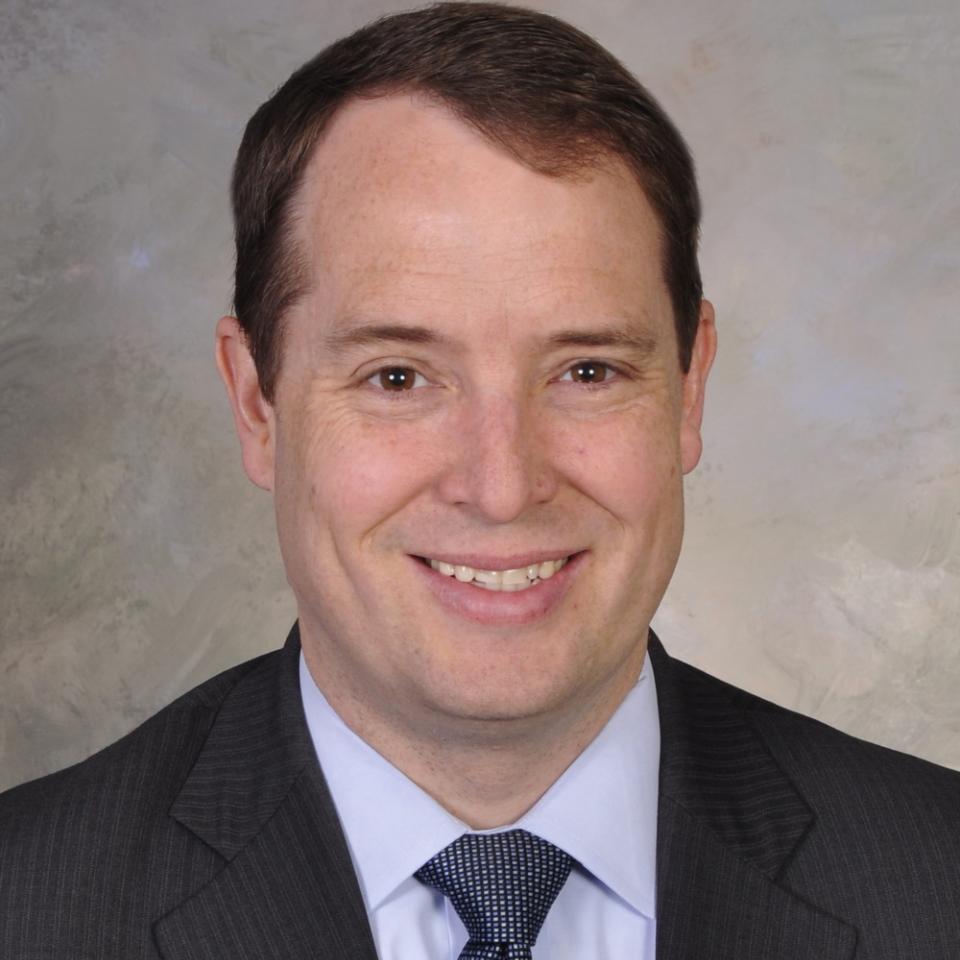
David K. Vawdrey, PhD

Adam Wilcox, PhD

Linying Zhang, PhD